Team
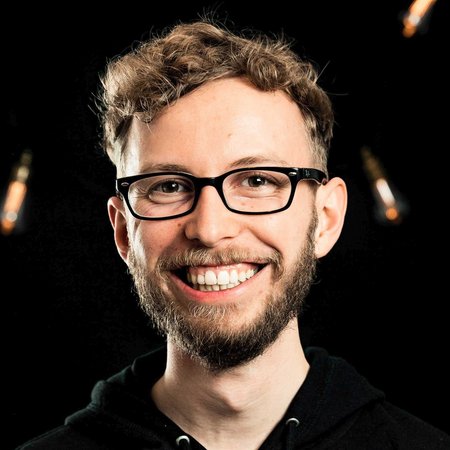
Academic Staff
M.Sc. Jonathan Berrisch
- Room:
- R11 T07 C12
- Phone:
- +49 201 18-36209
- Email:
- jonathan.berrisch (at) uni-due.de
- Homepage:
- https://berrisch.biz/
Publications:
- Berrisch, Jonathan; Ziel, Florian: Multivariate probabilistic CRPS learning with an application to day-ahead electricity prices. In: International Journal of Forecasting (2024). doi:10.1016/j.ijforecast.2024.01.005Full textCitationAbstractDetails
This paper presents a new method for combining (or aggregating or ensembling) multivariate probabilistic forecasts, considering dependencies between quantiles and marginals through a smoothing procedure that allows for online learning. We discuss two smoothing methods: dimensionality reduction using Basis matrices and penalized smoothing. The new online learning algorithm generalizes the standard CRPS learning framework into multivariate dimensions. It is based on Bernstein Online Aggregation (BOA) and yields optimal asymptotic learning properties. The procedure uses horizontal aggregation, i.e., aggregation across quantiles. We provide an in-depth discussion on possible extensions of the algorithm and several nested cases related to the existing literature on online forecast combination. We apply the proposed methodology to forecasting day-ahead electricity prices, which are 24-dimensional distributional forecasts. The proposed method yields significant improvements over uniform combination in terms of continuous ranked probability score (CRPS). We discuss the temporal evolution of the weights and hyperparameters and present the results of reduced versions of the preferred model. A fast C++implementation of the proposed algorithm is provided in the open-source R-Package profoc on CRAN.
- Berrisch, Jonathan; Narajewski, Michał; Ziel, Florian: High-resolution peak demand estimation using generalized additive models and deep neural networks. In: Energy and AI, Vol 13 (2023), p. 100236. doi:10.1016/j.egyai.2023.100236Full textCitationDetails
- Berrisch, Jonathan; Pappert, Sven; Ziel, Florian; Arsova, Antonia: Modeling volatility and dependence of European carbon and energy prices. In: Finance Research Letters, Vol 52 (2023), p. 103503. doi:10.1016/j.frl.2022.103503Full textCitationDetails
- Berrisch, Jonathan; Ziel, Florian: CRPS learning. In: Journal of Econometrics, Vol 237 (2023), p. 105221. doi:10.1016/j.jeconom.2021.11.008Full textCitationAbstractDetails
Combination and aggregation techniques can significantly improve forecast accuracy. This also holds for probabilistic forecasting methods where predictive distributions are combined. There are several time-varying and adaptive weighting schemes such as Bayesian model averaging (BMA). However, the quality of different forecasts may vary not only over time but also within the distribution. For example, some distribution forecasts may be more accurate in the center of the distributions, while others are better at predicting the tails. Therefore, we introduce a new weighting method that considers the differences in performance over time and within the distribution. We discuss pointwise combination algorithms based on aggregation across quantiles that optimize with respect to the continuous ranked probability score (CRPS). After analyzing the theoretical properties of pointwise CRPS learning, we discuss B- and P-Spline-based estimation techniques for batch and online learning, based on quantile regression and prediction with expert advice. We prove that the proposed fully adaptive Bernstein online aggregation (BOA) method for pointwise CRPS online learning has optimal convergence properties. They are confirmed in simulations and a probabilistic forecasting study for European emission allowance (EUA) prices.
- Berrisch, Jonathan; Ziel, Florian: Distributional modeling and forecasting of natural gas prices. In: Journal of Forecasting, Vol 41 (2022), p. 1065-1086. doi:10.1002/for.2853Full textCitationDetails